PhysAvatar: Learning the Physics of Dressed 3D Avatars
from Visual Observations
- * Yang Zheng 1
- * Qingqing Zhao 1
- Guandao Yang 1
- Wang Yifan 1
- Donglai Xiang 2
- Florian Dubost 3
- Dmitry Lagun 3
- Thabo Beeler 3
- Federico Tombari 3 4
- Leonidas Guibas 1
- Gordon Wetzstein 1
- Stanford University1
- Carnegie Mellon University2
- Google3
- Technical University of Munich4
-
* Project Co-leads
We introduce PhysAvatar, a novel framework that combines inverse rendering with inverse physics to automatically estimate the shape and appearance of a human from multi-view video data along with the physical parameters of the fabric of their clothes. We adopt a mesh-aligned 4D Gaussian technique for spatio-temporal mesh tracking as well as a physically based inverse renderer to estimate the intrinsic material properties. PhysAvatar integrates a physics simulator to estimate the physical parameters of the garments using gradient-based optimization in a principled manner. These novel capabilities enable PhysAvatar to create high-quality novel-view renderings of avatars dressed in loose-fitting clothes under motions and lighting conditions not seen in the training data.
Method Overview
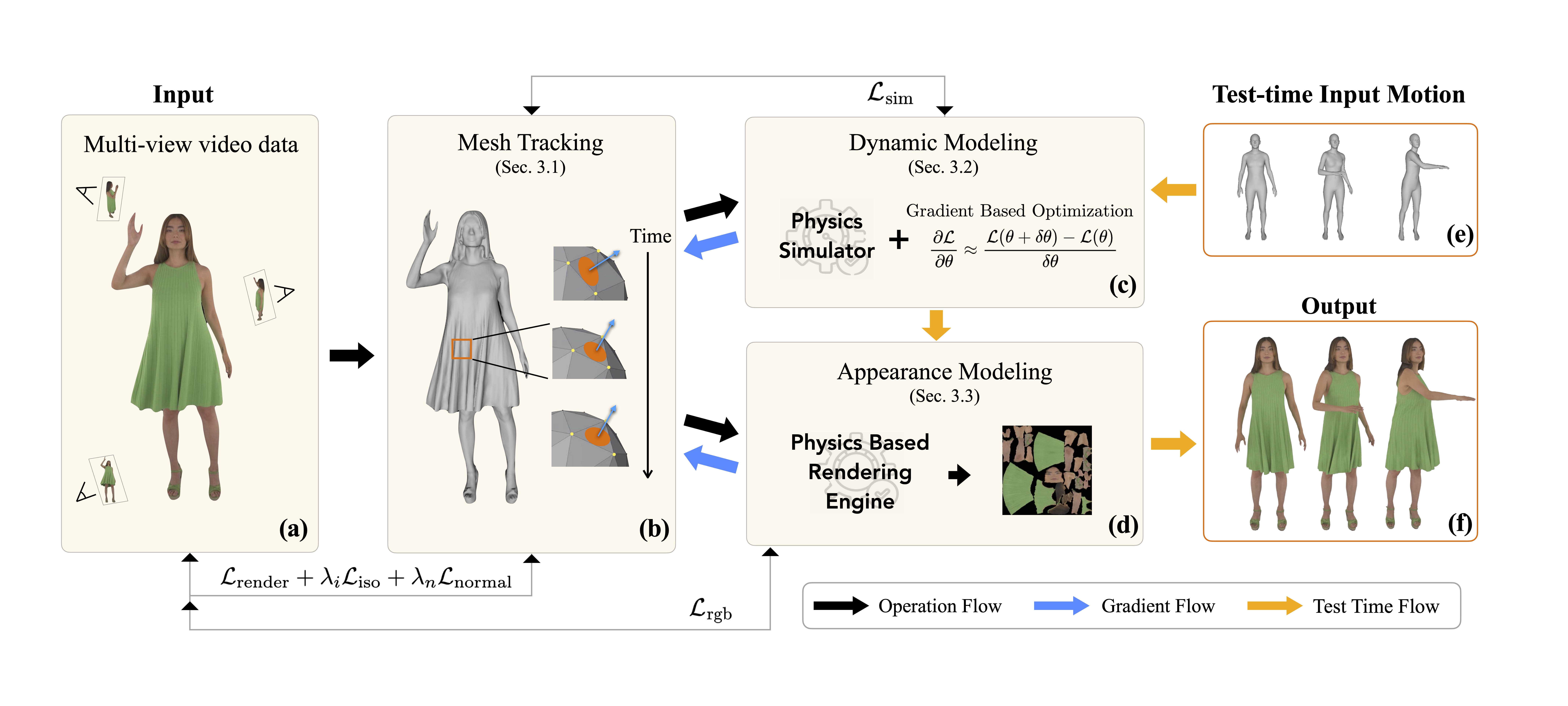
PhysAvatar takes multi-view videos and an initial mesh as input (a). We first perform (b) dynamic mesh tracking. The tacked mesh sequences are then used for (c) garment physics estimation with a physics simulator combined with gradient-based optimization; (d) and appearance estimation through physics-based differentiable rendering. At test time, (e) given a sequence of body poses (f), we simulate garment dynamics with the learned physics parameters and employ physics-based rendering to produce the final images.
Novel Motion
Novel Lighting
Redressing and Texture Painting
Video
Acknowledgements
We thanks Jiayi Eris Zhang for the discussions, and open source projects like Dynamics Gaussian Splatting, Codim-IPC and Mitsuba 3.
This material is based on work that is partially funded by an unrestricted gift from Google, Samsung, an SNF Postdoc Mobility fellowship, ARL grant W911NF-21-2-0104, and a Vannevar Bush Faculty Fellowship.
Citation
@inproceedings{PhysAavatar24,
title={PhysAvatar: Learning the Physics of Dressed 3D Avatars from Visual Observations},
author={Yang Zheng and Qingqing Zhao and Guandao Yang and Wang Yifan and Donglai Xiang and Florian Dubost and Dmitry Lagun and Thabo Beeler and Federico Tombari and Leonidas Guibas and Gordon Wetzstein}
journal={arxiv},
year={2024}
}
Any opinions, findings, and conclusions or recommendations expressed in this material are those of the author(s) and do not necessarily reflect the views of Google.